Abstract
Recently, gait patterns of autistic children are of interest in the gait community in order to identify significant gait parameter namely the three-dimensional (3D) gait features. The development of gait patterns via assessing gait deviations in autistic children can help clinicians and researchers to differentiate gait pattern abnormality in diagnosing, clinical decision-making and planning. Understanding the characteristics and identifying gait pattern is essential in order to distinguish normal as well as abnormal gait pattern. Hence in this research, the application of machine learning approach specifically Neural Network (NN) and Support Vector Machine (SVM) along with Singular Value Decomposition (SVD) and Linear Discriminant Analysis (LDA) as feature extractions are evaluated and validated in discriminating gait features between normal subjects and autistic children. Gait features of 32 normal and 12 autistic children were recorded and analyzed using VICON motion analysis system and force platform during normal walking. Here, twenty-one gait features describing the three types of gait characteristics namely basic, kinetic and kinematic in these children are extracted. Next, these three category gait parameters acted as inputs to both classifiers. The performance of NN and SVM in classifying the gait patterns between autistics and normal children as classifier are evaluated. The ability of SVM as classifier are investigated using three different kernel functions specifically linear, polynomial, and radial basis. In addition, the classifiers performance is validated by computing both values of sensitivity and specificity. Results showed that LDA as feature extraction has the highest accuracy with kinematic parameters as gait features along with polynomial function as kernel for the SVM classifier with 100% accuracy. This finding proven that LDA is suitable as feature extraction and SVM is indeed apt as gait classifier in classifying the gait pattern autistics and normal children.
Metadata
Item Type: | Thesis (Masters) |
---|---|
Creators: | Creators Email / ID Num. Ilias, Suryani UNSPECIFIED |
Contributors: | Contribution Name Email / ID Num. Thesis advisor Md Tahir, Nooritawati UNSPECIFIED |
Divisions: | Universiti Teknologi MARA, Shah Alam > Faculty of Electrical Engineering |
Programme: | Master of Science |
Keywords: | autism, children, gait |
Date: | 2018 |
URI: | https://ir.uitm.edu.my/id/eprint/79396 |
Download
![[thumbnail of 79396.pdf]](https://ir.uitm.edu.my/style/images/fileicons/text.png)
79396.pdf
Download (184kB)
Digital Copy
Physical Copy

ID Number
79396
Indexing
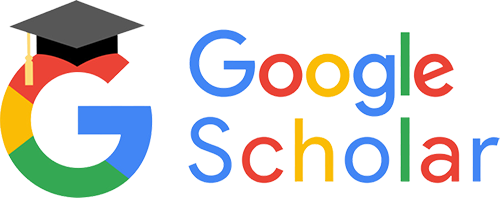
