Abstract
A system identification in control engineering field is a mathematical model based on dynamic properties of the system. Previous researches have established that system identification received a significant attention and interest in broad disciplines due to the theoretical advancement, good predictive model, and performance accuracy. Existing research recognises the critical role played by the accurate nonlinear modelling for distillation column during the essential oil extraction process. The Nonlinear Auto Regressive with exogenous input (NARX) is one of the most current widely used because of highly efficient, simple, accurate and outstanding results in the actual nonlinear system applications. In recent years, there has been an increasing amount of optimisation modelling research on time-varying nonlinear modelling in the actual nonlinear system applications which hasn't been explored for Steam Distillation Pilot Plant (SDPP) to date. The appropriate choice of the input signals for the nonlinear system is essential to obtain a good performance in the system identification since the widely used Pseudo Random Binary Signal (PRBS) was reported as an insufficient to reveal the nonlinearity behaviour. This study proposes a system identification of SDPP using NARX model. The model structure selection of polynomial NARX had been focused on Binary Particle Swarm Optimisation (BPSO) algorithm. The proposed NARX-based BPSO algorithm was implemented for both time-varying water and steam temperature from the SDPP. The time-varying data from this nonlinear system employed two perturbation input signals, PRBS and Multi-level Pseudo Random Sequence (MPRS). Several model's selection criteria such as Akaike Information Criterion (AIC), Final Prediction Error (FPE), and Model Descriptor Length (MDL) were investigated. Prior to analysing the efficiency of SDPP nonlinear models, the NARX-based BPSO model was checked based on the model validation. The performance comparisons were measured between these two PRBS and MPRS perturbation input signals for both water and steam temperature modelling. The findings reported the NARX models for water and steam temperature models demonstrated excellent outcomes through BPSO algorithm structure selection. The combination of BPSO algorithm and NARX model on the time-varying process produced higher accuracy (99.9999 %) with low Mean Square Error (MSE) (0.01 to 0.009), revealed the real feature of the nonlinear data and effectively captivated the nonlinear properties of the dynamic response. In addition, the results of this study 9indicated that the selection of MPRS perturbation input signal for the water (3 parameters) and steam temperature (3 parameters) dataset of the SDPP contributed to better modelling performance with the least number of parameters in the output models compared with PRBS signal (5 to 7 parameters).
Metadata
Item Type: | Thesis (PhD) |
---|---|
Creators: | Creators Email / ID Num. Hambali, Najidah 2015454752 |
Contributors: | Contribution Name Email / ID Num. Thesis advisor Rahiman, Mohd Hezri Fazalul UNSPECIFIED |
Subjects: | Q Science > QA Mathematics > Mathematical statistics. Probabilities Q Science > QA Mathematics > Analysis > Analytical methods used in the solution of physical problems > System analysis. State-space methods |
Divisions: | Universiti Teknologi MARA, Shah Alam > Faculty of Electrical Engineering |
Programme: | Doctor of Philosophy (Electrical Engineering) |
Keywords: | System identification, NARX modelling, binary particle |
Date: | 2019 |
URI: | https://ir.uitm.edu.my/id/eprint/68729 |
Download
![[thumbnail of 68729.pdf]](https://ir.uitm.edu.my/style/images/fileicons/text.png)
68729.pdf
Download (18kB)
Digital Copy
Physical Copy

ID Number
68729
Indexing
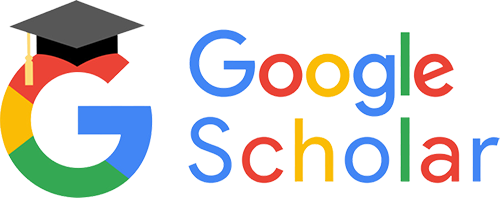
