Abstract
Air is a mixture comprising a group of almost continuous concentrations of gasses and a group of concentrations that differ in both space and time. The importance of tracking and regulating air quality is, in particular, mandatory in today's era of growth. Air pollution has created bad effects on many sides, particularly on living things. The Markov Chain model was proposed to forecast the Air Pollution Index, which is a stochastic model that relies on the previous state in time. It also contains the state transition matrix and the stationary probability distribution for model growth. Besides, the Linear Interpolation method was used to fill the incomplete Air Pollution Index data values that were obtained. The result revealed that the model had successfully established a valuable method for estimating potential states of the Air Pollution Index as the result was consistent and precise. The Markov Chain model is also the best choice for estimating air quality and very well determining the long-term spread of pollution.
Metadata
Item Type: | Student Project |
---|---|
Creators: | Creators Email / ID Num. Aasi @ Aziz, Muhammad Asyraf 2019312275 |
Subjects: | Q Science > QA Mathematics > Probabilities T Technology > TD Environmental technology. Sanitary engineering > Air pollution and its control |
Divisions: | Universiti Teknologi MARA, Perlis > Arau Campus > Faculty of Computer and Mathematical Sciences |
Programme: | Management Mathematics |
Keywords: | Forecasting Air Pollution Index ; Markov Chain ; Linear Interpolation |
Date: | 29 July 2021 |
URI: | https://ir.uitm.edu.my/id/eprint/49211 |
Download
![[thumbnail of 49211.pdf]](https://ir.uitm.edu.my/style/images/fileicons/text.png)
49211.pdf
Download (176kB)
Digital Copy
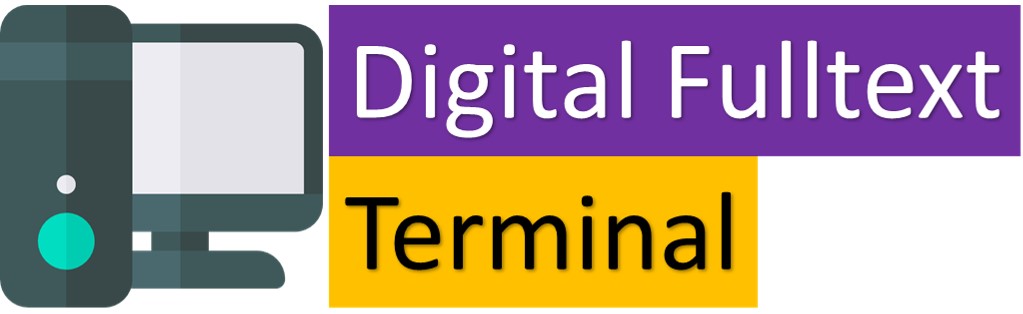
Physical Copy
ID Number
49211
Indexing
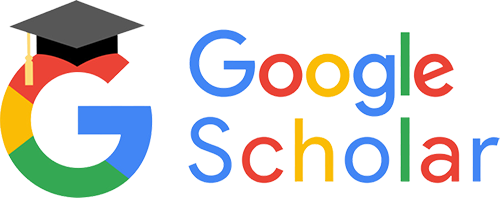
