Abstract
Mangroves are ecosystems that grow and dominate the coastal areas of tropical and sub-tropical regions across the world. Mangroves provide crucial socio-economic supports and play a pivotal role in ecological ecosystem functions especially in offsetting an excess of carbon from the atmosphere. Despite the crucial roles provided by mangroves, these ecosystems have degraded at an alarming rate mainly due to climate change and anthropogenic activities. Given the important of mangroves in offsetting excessive carbon, effective inventories and monitoring methods have prompted this research into supplementing ground field surveys with the information from satellite remote sensing data. The objectives of this study were i) to determine level of knowledge and awareness among local communities towards mangrove trends and status in the study area ii) to analyse land use/cover changes of mangroves using a time series of Landsat images iii) to establish relationships between mangroves stand parameters and spectral reflectance recorded by Landsat 8 OLI data iv) to develop predictive models for estimating the above ground biomass (AGB) using the appropriate combinations of ground data and Landsat 8 images v) to produce thematic maps of biomass and carbon sequestration of mangrove forests based on predictive model application. The field-measured AGB and carbon stocks were related to an individual Landsat 8 Operation Land Imager (OLI) bands and 11 vegetation indices using multiple regression analysis. The good models were selected based on stepwise and backward elimination methods where the coefficient of determination (R2), adjusted R2 (adj. R2), standard error of estimate (SEE), roots mean squared error (RMSE), correlation index square (I2) and Mallows’ prediction criterion (Cp) were evaluated in model development and validation. From the regression analysis, it was found that the most promising model provides moderately good prediction of about 56% of the variability of the AGB and carbon stocks with RMSE values of 48.04 Mg/ha and 22.24 Mg/ha, respectively. It was found that the application of Landsat 8 OLI data offer cost effective and wide area coverage for the estimation of AGB and carbon stocks of mangroves with moderate accuracy. The combination use of multi-date Landsat imagery, post-classification technique and Geographic Information System (GIS) have provided useful tools to map mangrove changes and their statistics. This information is necessary in providing in-depth understanding on the general processes of changes and contributing to the effective mangrove management for sustainable resources. The implementation of appropriate policy for mangroves, with the knowledge and awareness of local community can spur for a better management and utilization of mangroves resources in the study area. In addition, the information from this thesis may provide useful tool for resource planners and mangroves-related agencies in making resource forecasts, and assist in the resource planning and management towards the sustainability of mangrove ecosystem.
Metadata
Item Type: | Thesis (PhD) |
---|---|
Creators: | Creators Email / ID Num. Tengku Hashim, Tengku Mohd Zarawie 2011238304 |
Contributors: | Contribution Name Email / ID Num. Thesis advisor Suratman, Mohd Nazip (Prof. Dr. ) UNSPECIFIED |
Subjects: | Q Science > QE Geology > Dynamic and structural geology > Geochemistry T Technology > TP Chemical technology > Fuel > Biomass |
Divisions: | Universiti Teknologi MARA, Shah Alam > Faculty of Applied Sciences |
Programme: | Doctor of Philosophy in Science – AS 990 |
Keywords: | carbon, biomass, Kedah |
Date: | 2021 |
URI: | https://ir.uitm.edu.my/id/eprint/46486 |
Download
![[thumbnail of 46486.pdf]](https://ir.uitm.edu.my/style/images/fileicons/text.png)
46486.pdf
Download (320kB)
Digital Copy
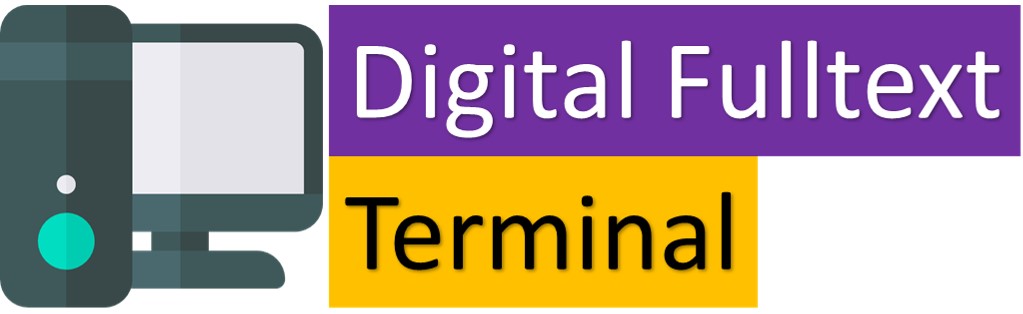
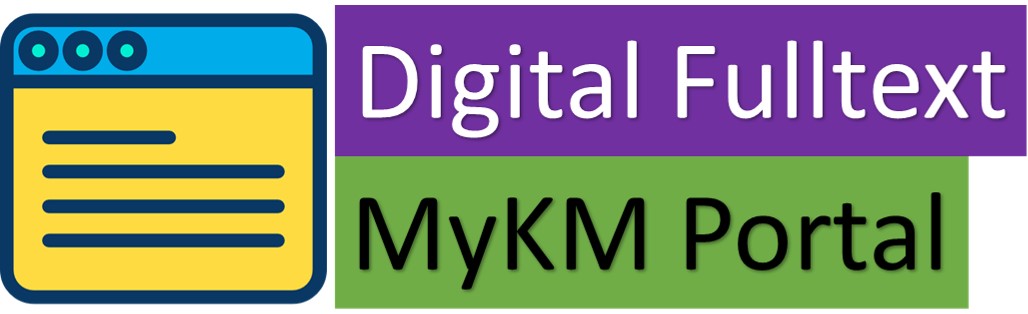
Physical Copy
ID Number
46486
Indexing
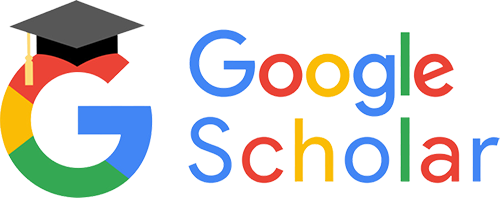
