Abstract
This thesis presents new and novel linear and nonlinear system identification for a steam distillation essential oil extraction system. The system identification is initialized with a step test to estimate the system dynamics before continuing with PRBS perturbations. Four PRBS tests have been presented in this thesis, where the first three were collected under different PRBS settings and the fourth was collected without low-pass filter. The collected data were then pre-processed and analyzed to evaluate their power spectral densities, correlation functions, distributions and sampling intervals. In the linear system identification approach, the ARX and ARMAX model structures have been used to model the system dynamic. The selection of model order for both structures was made based on information criterions such as NSSE, AIC, FPE and MDL. The selected ARX and ARMAX structures were estimated and validated. The performances of both models were then further assessed by noise variation tests and OSA evaluation tests. Results have shown that both models are good enough to model the system dynamic with the best fit of no less than 90%. In general, the ARX model with AIC selected model order is the most preferable as compared to the rest. Subsequently, in the nonlinear system identification, the NNARX model structure was used to model the system dynamic. Several optimizations on the neural network training have been performed and several regularizations of the training have been considered. Similar investigations have been carried out in nonlinear identification as done in its linear counterpart. Based on comparison between all above models, the NNARX that coupled with suitable regularisations have outperformed the linear models. Even though the linear models are sufficient for this system, the nonlinear model can represent the system better. The selected final model is the NNARX model structure with MDL model order selection criterion. The model was trained with LMA under lxlO"4 weight decay regularisation, 5 hyperbolic-tangent hidden neurons and one linear output neuron.
Metadata
Item Type: | Thesis (PhD) |
---|---|
Creators: | Creators Email / ID Num. Fazalul Rahiman, Fazalul Rahiman 2004360861 |
Contributors: | Contribution Name Email / ID Num. Thesis advisor Taib, Mohd Nasir UNSPECIFIED |
Subjects: | Q Science > QD Chemistry > Physical and theoretical chemistry T Technology > TP Chemical technology > Chemical engineering > Special processes and operations > Extraction |
Divisions: | Universiti Teknologi MARA, Shah Alam > Faculty of Electrical Engineering |
Programme: | Doctor of Philosophy |
Keywords: | Essential oil extraction, nonlinear system identification, linear counterpart |
Date: | 2009 |
URI: | https://ir.uitm.edu.my/id/eprint/41297 |
Download
![[thumbnail of 41297.pdf]](https://ir.uitm.edu.my/style/images/fileicons/text.png)
41297.pdf
Download (185kB)
Digital Copy
Physical Copy

ID Number
41297
Indexing
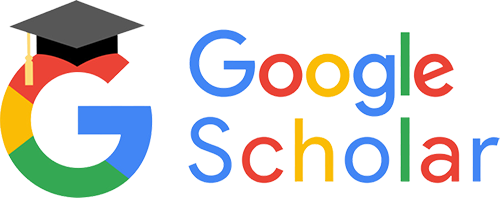
