Abstract
Automated vision recognition has been widely implemented for various fields such as automobiles, manufacturing, medical, agricultural sector, etc. However, automation recognition specifically in oil palm or scientifically known as Elaeis Guineensis industry is still lacking. To the best of our knowledge, automatic detection device for nutrition-lacking disease based on appearance of symptoms on leaf surfaces is unavailable since at present, the disease is inspected by human experts depending on the knowledge and experience possessed. Hence, this thesis proposed to automate the nutritional disease detection due to nutritional deficiencies namely nitrogen, potassium and magnesium instead of manual visual recognition. This is because automation process is necessary to lessen error and reduce cost due to human experts as well as to increase speed of disease detection. Generally, the proposed automation disease detection of oil palm involves three modules namely feature extraction based on image processing technique, statistical analysis as feature selection and classification based on artificial intelligence. Firstly, the diseased-frond leaf surface image is captured at ambient environment. This uncontrolled processing environment approach implemented for disease detection based on leaf surface appearance is considered new and can be regarded as significant contribution in this research field. Next, the captured leaf image is transmitted to the host computer database for further processing. Further, the processor formulates its judgment through machine learning that is able to infer decision similar to human thinking. Here, the performances of several machine learning classifiers are compared. Once the processing stage is completed, the image will be retrieved online through the portable device of Apple's Operating System (iOS) (ipod/iphone/ipad tablet) technology. Results demonstrated that support vector machine (SVM) of radial basis function (RBF) outperformed other classifiers in recognizing the disease types from the leaf surface. Furthermore, it was also found that SVM-RBF is the most suitable method for classifying the disease in terms of accuracy and processing speed. Feature selection via Analysis of Variance (ANOVA) and Multiple Comparison Procedure (MCP) enhanced classifier prediction capability, thus resemble original features as closest as possible without compromising the accuracy rate. Results revealed that higher recognition rates attained with classification based on SVM-RBF along with appropriate feature selection that yields accuracy from 91.11% to 91.81 %.
Metadata
Item Type: | Thesis (PhD) |
---|---|
Creators: | Creators Email / ID Num. Hairuddin, Muhammad Asraf 2010213996 |
Subjects: | Q Science > QD Chemistry > Analytical chemistry R Medicine > RE Ophthalmology > Refraction and errors of refraction and accommodation |
Divisions: | Universiti Teknologi MARA, Shah Alam > Faculty of Electrical Engineering |
Programme: | Doctor of Philosophy |
Keywords: | Automated Vision Recognition; Classifying Nutrient Deficiencies; Elaeis Guineensis Leaf |
Date: | July 2014 |
URI: | https://ir.uitm.edu.my/id/eprint/16248 |
Download
![[thumbnail of 16248.pdf]](https://ir.uitm.edu.my/style/images/fileicons/text.png)
16248.pdf
Download (149kB)
Digital Copy
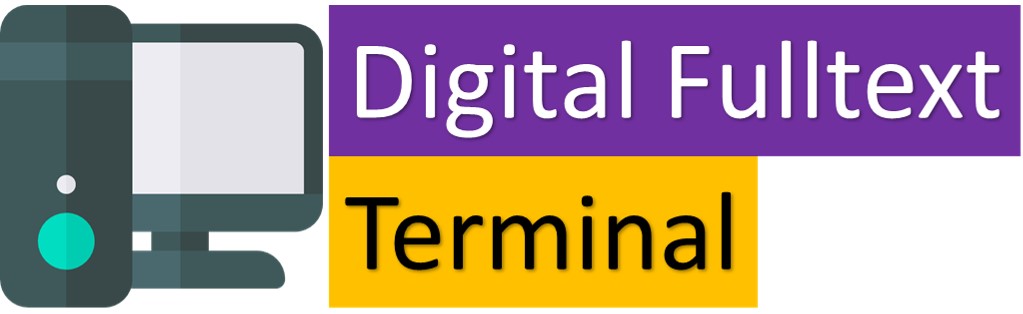

Physical Copy

ID Number
16248
Indexing
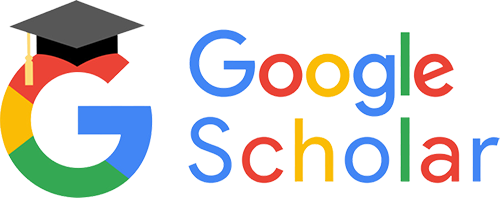
