Abstract
Medium Density Fiberboard (MDF) is an alternative to solid wood used in furniture industries. As an engineered wood, MDF needs to establish the strength level to guarantee its quality. The test procedures for mechanical and physical properties of MDF should conform to a specified standard, prior to releasing processed fiberboards for manufacturing. These tests are costly for they involve a high amount of resources, especially to research institutions. The primary aim of this research is to reduce testing time of three lengthy procedures; namely, 24-hour thickness swelling, 24-hour water absorption and 48-hour moisture content. An intelligent predictive model will replace the lengthy procedures by predicting the properties using known fiberboard characteristics. Back-propagation algorithm is a training method widely used in a multilayer perceptron Neural Network model. It optimizes random values for network weights and biases. However, the result normally faces local optima problems. This situation can be solved
by embedding Genetic Algorithm (GA) in the network to replace back-propagation method. GA uses its reproduction capability to evolve from local minima scenario through crossover and mutation operators. Crossover and mutation activities contribute towards the quality of offspring and the operators’ probability rates control the chances of reproduction. Nevertheless, a fixed probability rates will cause convergence to beslower; for the activities constantly take place regardless of the changes in population fitness. Therefore, adjustment for suitable rates is important. Adaptive mechanism adapts the best parameters of current generation for optimum performance in the next generation. A variable rate adapts with the current population environment and therefore increases the searching ability. A new adaptive mechanism is done by scanning through the fitness mean and median of the population using the prediction error. Through rank selection technique, the chromosomes are sorted based on the fitness function to learn about the population of current generation. The adaptive mechanism on GA has allowed earlier convergence as compared to the ordinary GA with fixed probability rates. Comparison analysis shows the cost difference using the new method and the existing method to prove method efficiency. This research discusses three predictive models using multilayer perceptron NN with different optimizers. The first model uses BP; the second is hybrid GA-NN model; and finally a hybrid GA-NN model with an adaptive mechanism. The result has reduced time taken and experimental cost for the lengthy testing procedures. Consequently, during the experimental tests, pilot plants will only need to carry out tests, which consume minimum time to complete. The novelty of the research is a hybrid GA-NN model with new adaptive mechanism, along with contributions of reduction in experimental time and costs for MDF testing.
Metadata
Item Type: | Thesis (PhD) |
---|---|
Creators: | Creators Email / ID Num. Sh. Ismail, Faridah UNSPECIFIED |
Subjects: | |
Divisions: | Universiti Teknologi MARA, Shah Alam > Faculty of Computer and Mathematical Sciences |
Programme: | Doctor of Philosophy |
Keywords: | Neural network; Physical properties; Oil palm; Fiberboard |
Date: | 2015 |
URI: | https://ir.uitm.edu.my/id/eprint/15905 |
Download
![[thumbnail of TP_FARIDAH SH. ISMAIL CS 15_5.PDF]](https://ir.uitm.edu.my/style/images/fileicons/text.png)
TP_FARIDAH SH. ISMAIL CS 15_5.PDF
Download (2MB)
Digital Copy
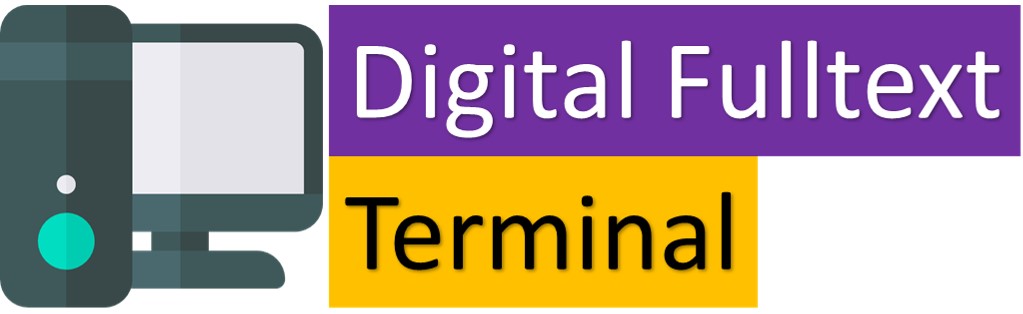
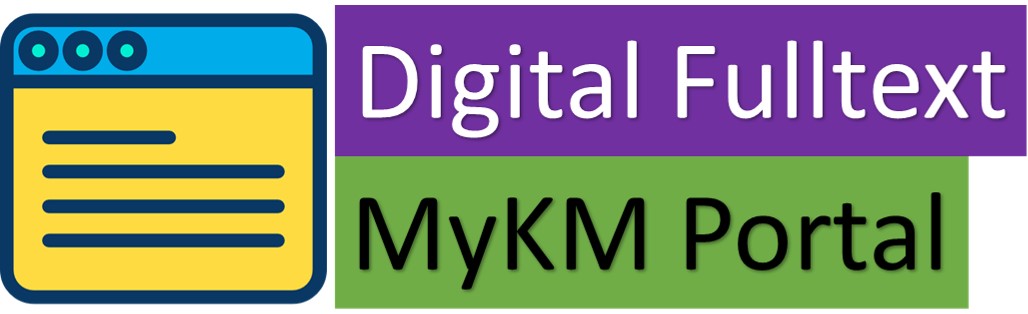
Physical Copy

ID Number
15905
Indexing
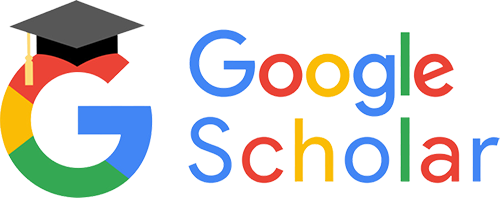
