Abstract
Conventional water quality estimation of the river requires in situ measurements and laboratory analysis which are extremely demanding in terms of time consuming and cost. In remote sensing technology, water turbidity can be estimated by the capability of remotely sensed spectral properties such as by using Landsat 8 OLI. Nowadays, a local regression approach of Geographically Weighted Regression (GWR) is widely used to improve the global approach such as linear regression method. Thus, the aim of this study is to assess satellite based water turbidity of Landsat 8 OLI with in situ measurement in Perlis River during Southwest Monsoon by using GWR technique. The objectives are to estimate water turbidity from Landsat OLI and to assess the estimated water turbidity with in situ measurement using GWR. The estimation of water turbidity from Landsat 8 is done by the extraction of satellite DN and spectral reflectance of Ultra Blue, Green, and Red bands a based on water sampling locations respectively. With 30 samples of water sampling, the assessment of satellite based water turbidity is carried out using GWR. The result shows strong correlation for both local relationship between in situ turbidity data and (I) DN of green band (R2 = 0.546) and spectral reflectance of Red Band (R2 =0.745). Thus, the Landsat DN of green band and spectral reflectance of Red Band are suitable to estimate water turbidity. The finding of this study shows, the local relationship between satellite based and in situ water turbidity can be determined. Thus, remotely sensed of spectral band is possible to be utilized for water turbidity estimation.
Metadata
Item Type: | Thesis (Degree) |
---|---|
Creators: | Creators Email / ID Num. Abdul Rahman, Nurul Adila UNSPECIFIED |
Subjects: | G Geography. Anthropology. Recreation > G Geography (General) > Geographic information systems G Geography. Anthropology. Recreation > GB Physical geography > Hydrology. Water > Turbidity T Technology > TL Motor vehicles. Aeronautics. Astronautics > Artificial satellites in navigation |
Divisions: | Universiti Teknologi MARA, Perlis > Arau Campus > Faculty of Architecture, Planning and Surveying |
Keywords: | Geographically Weighted Regression (GWR) ; Satellite based ; Water turbidity |
Date: | February 2018 |
URI: | https://ir.uitm.edu.my/id/eprint/19131 |
Download
TD_NURUL ADILA ABDUL RAHMAN AP R 18_5.pdf
Download (242kB) | Preview
Digital Copy
Physical Copy
ID Number
19131
Indexing
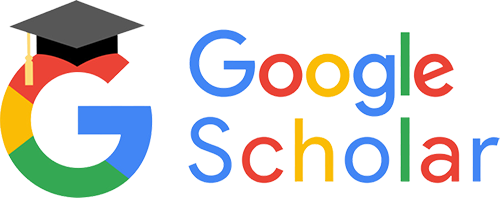
