Abstract
Electroencephalogram (EEG) is a non-invasive approach for measuring brainwaves applied extensively in cognitive studies. Intelligence, which is commonly gauged as intelligence quotient (IQ) is one of the human potential ability that originates from cognitive functioning of the brain. Recent researches have shown that correlation exists between EEG and IQ. Furthermore, various advanced studies on the EEG signal are conducted using advanced computation methods. However, a systematic approach for IQ classification based on brainwaves and intelligent modelling technique has yet to be studied. Hence, this thesis proposed a practical and systematic approach to develop IQ classification model via artificial neural network (ANN) based on EEG sub-band features which then, can be related with brain asymmetry (BA) and learning style (LS). The protocols involved EEG recording during resting with eyes closed and answering the conventional psychometric test. Fifty subjects of UiTM students are divided into three IQ levels based on the IQ scores from Raven’s Progressive Matrices as the control group. Power ratio (PR) and spectral centroid (SC) features of Theta, Alpha and Beta are extracted from left prefrontal cortex EEG signals. Then, the distributions of sub-band features are examined for each IQ level. Cross-relational studies are also done between IQ and other cognitive abilities, which are brain asymmetry and learning style based on EEG features. Further, IQ classification models comprising of inputs based on PR and SC features (Model A and Model B) are developed using multilayer feedforward network. Findings from this research showed that sub-band PR and SC features are indeed correlated with IQ. Consequently, the
network models yielded low mean squared error (MSE) and fulfilled the correlation requirements in classifying IQ levels. In cross-relational studies, findings have also revealed that PR and SC in relaxed closed-eyes state reflect the relationship between intelligence and other cognitive abilities. The results showed that different balanced states of the brain and learning styles can be mapped to distinct IQ levels using the developed models. Results also demonstrated that high IQ is obtained when subjects maintain relatively balanced hemispheric control. Additionally, results also revealed that medium and high IQ levels are capable of utilising four learning styles compared to low IQ level where only three learning styles were utilised. In conclusion, this research has proven that IQ level classification via EEG and ANN modelling is successful specifically the PR and SC features at resting EEG that can be considered as a stable biological marker in relation to cognitive performance. In addition, the study also confirmed that left hemisphere of the frontal region is adequate for IQ recognition.
Metadata
Item Type: | Thesis (PhD) |
---|---|
Creators: | Creators Email / ID Num. Jahidin, Aisyah Hartini 2011600522 |
Subjects: | Q Science > QA Mathematics > Instruments and machines > Electronic Computers. Computer Science > Neural networks (Computer science) |
Divisions: | Universiti Teknologi MARA, Shah Alam > Faculty of Electrical Engineering |
Programme: | Doctor of Philosophy |
Keywords: | Artificial neural network modelling; IQ classification; EEG signals |
Date: | 2015 |
URI: | https://ir.uitm.edu.my/id/eprint/15601 |
Download
![[thumbnail of 15601.pdf]](https://ir.uitm.edu.my/style/images/fileicons/text.png)
15601.pdf
Download (497kB)
Digital Copy
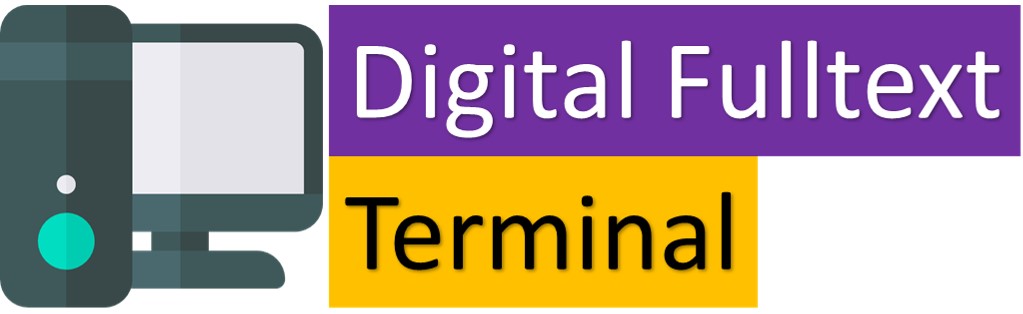

Physical Copy
ID Number
15601
Indexing
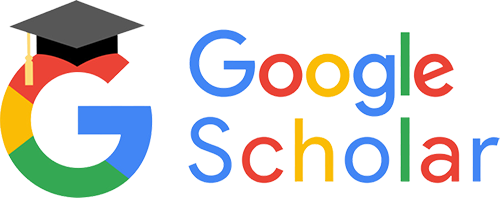
